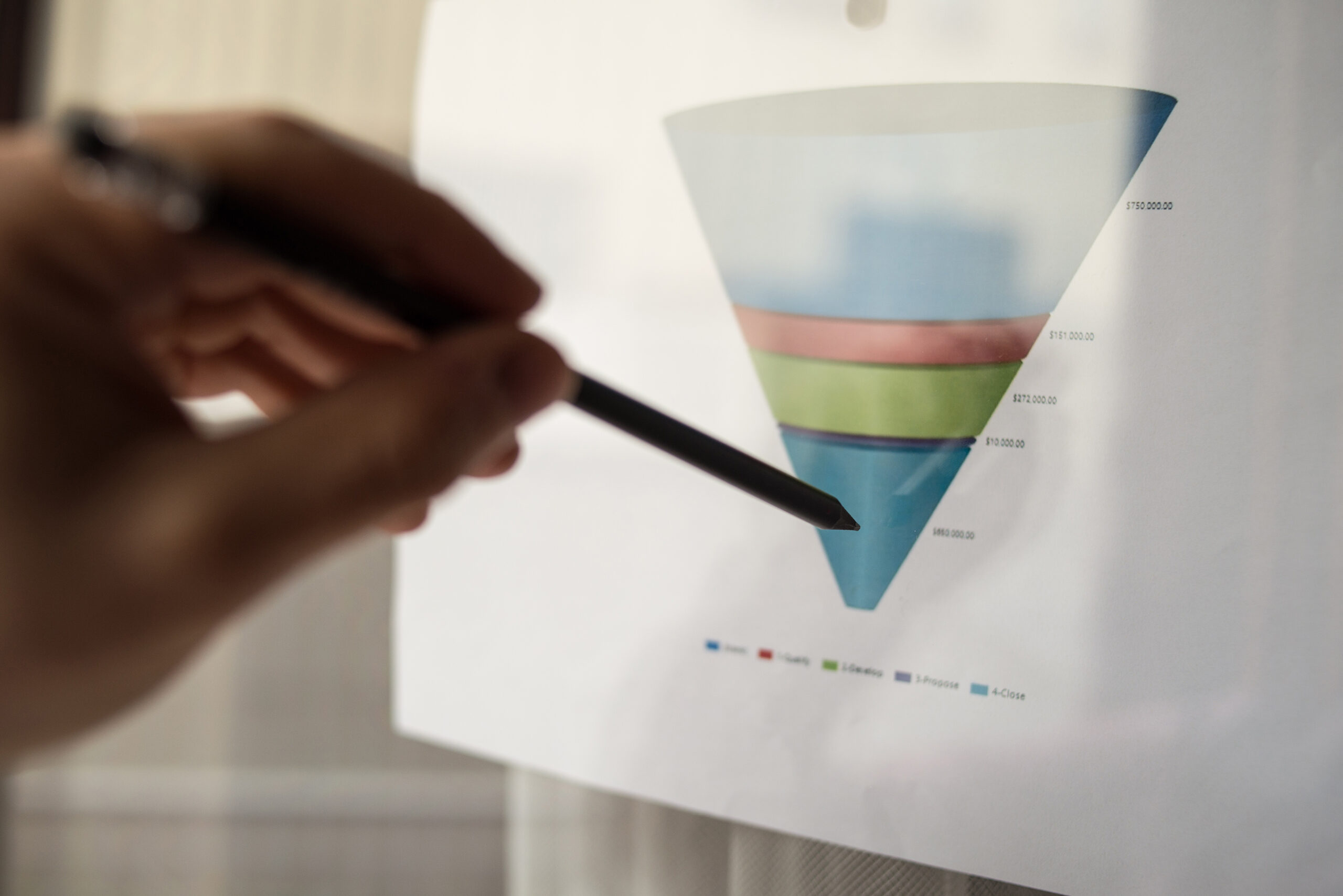
At every step in the marketing funnel, data can improve results
Published 11th Jan 2019When marketing to consumers, identifying prospective customers is only the beginning. Sending them solicitations is only a step in the process. Receiving a response from the customer doesn’t even mark the halfway point to a successful sale.
Those involved in sales are likely aware of the concept of the marketing funnel. At the top of the funnel is the awareness stage. There are lots of people there. Like all funnels, it narrows toward the bottom, where just a small percentage of the people aware of your product ever become customers. Along the way, there are stages, such as when customers engage with a company and evaluate alternatives, where some percentage of potential customers are lost.
Powerlytics recently worked with a direct-to-consumer non-bank lender to identify data that could improve the results of its marketing funnel at every step of the process. Using our proprietary data and the lender’s list of potential customers, we found dozens of variables that could do the job.
Our customer, which markets personal loans through direct mail, received 2.8 million loan applications in response to a campaign. Of those, about 1 million were approved for a loan, and of those that were approved, 177,000 told the lender they wanted to complete the transaction.
The number of people that actually received funds was even less than the number of people that said they wanted the money after they were approved. Just a fraction of the qualifying consumers accepted funds. In all, of the 2.8 million applications, the lender made just 141,000 loans, of which 8,500 defaulted.
The lender stood to save millions of dollars in wasted time and money by using data that would allow it to target consumers it was more likely to approve. And they could earn more if that targeting focused on people who were more likely to accept and less likely to default.
The Powerlytics database of financial information contains a comprehensive and anonymized financial view of over 200 million adults, down to the ZIP+4 level. This extreme granularity does not need to be gathered by the lender or reported by the consumer. The only data the lender must bring to the table is the consumer’s ZIP+4.
Through testing, 534 variables were identified that would have improved the lender’s results in the first stage by more than five percent, and 22 variables that have a 20 percent difference between those that were approved and not approved.
For example, applicants living in a ZIP+4’s in which more people reported self-employment were 33 percent more likely to be approved.
Another big indicator was how the immediate neighborhood of an applicant compared to the larger area around it. When Powerlytics examined applicants whose ZIP+4 had changes to non-wage income (that is, income reported that isn’t reported on a W2) when compared to the change to non-wage income of the larger zip code, we found a 254 percent difference in the likelihood that the lender would accept the application.
Non-wage income is often not received with the same consistency of picking up a pay check twice a month, so it often becomes an income stability metric. Our data showed that the more people in a ZIP+4 saw their non-wage income change, the more predictive it was in the next two stages of the funnel.
In other words, where there were big swings in non-wage income compared to surrounding areas, consumers were more likely to indicate they wanted the loan, and more likely to accept the funds.
We also examined differences in those that defaulted on their loans and those that did not. Again, variables that might seem to be counterintuitive were predictive of default. Our data showed that people living in ZIP Codes that saw a change to the number of people using a paid tax preparer were more likely to default.
Powerlytics data could be used to improve marketing funnels in other industries, such as credit cards, or it can be used to identify opportunities for cross-selling or upselling. That’s because different types of income, as well as other financial attributes of consumers (of which Powerlytics has over 200), can be highly predictive of consumer behavior. You just need the right data to make the prediction.
Read next
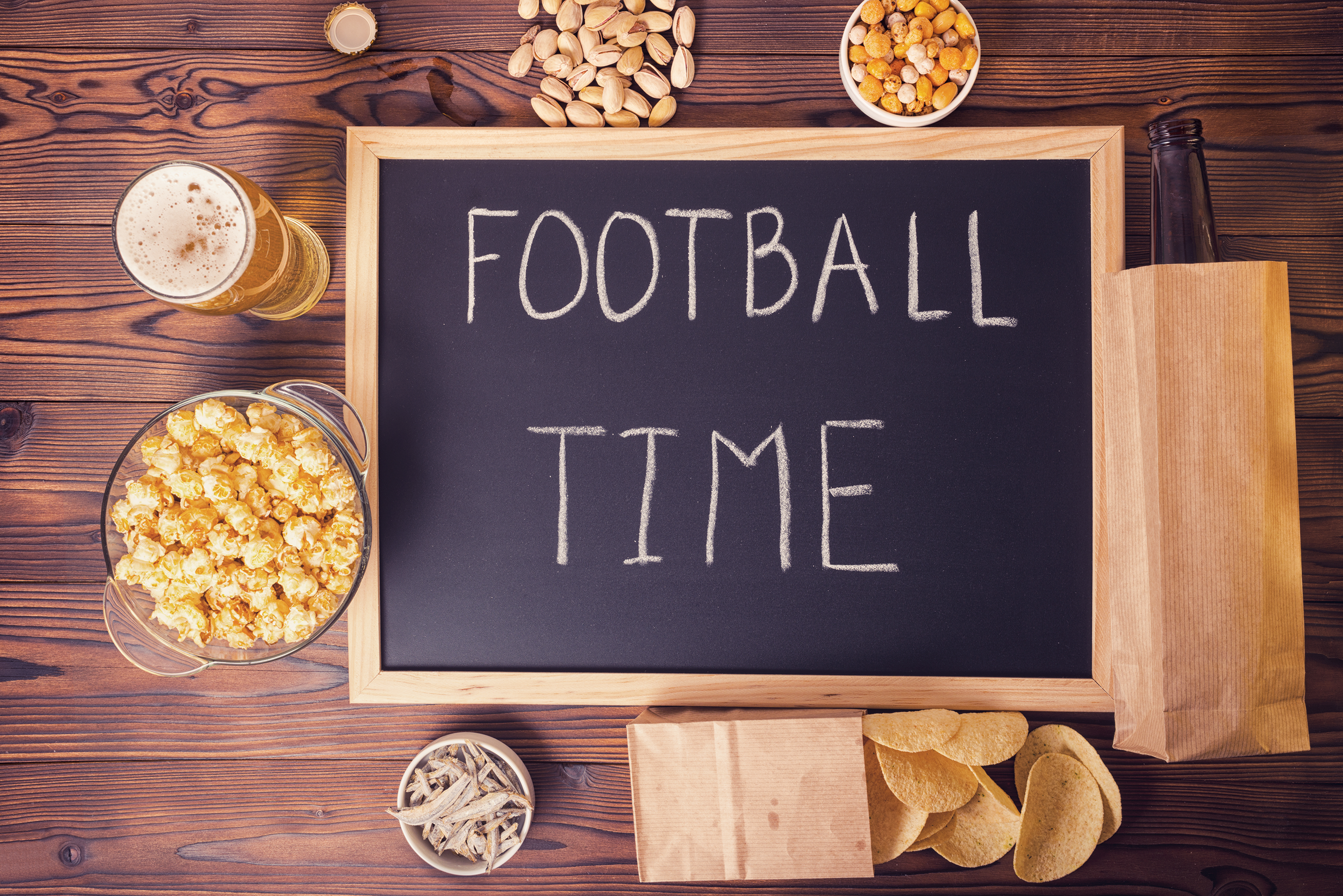
Published 14th Nov 2018
Predictive AnalyticsA snapshot of the economy behind Game Day
For huge swaths of the American public, Saturdays and Sundays in fall are national holidays. They’re days set aside to pile up the plate and watch football in a weekly ritual of...
Read Article