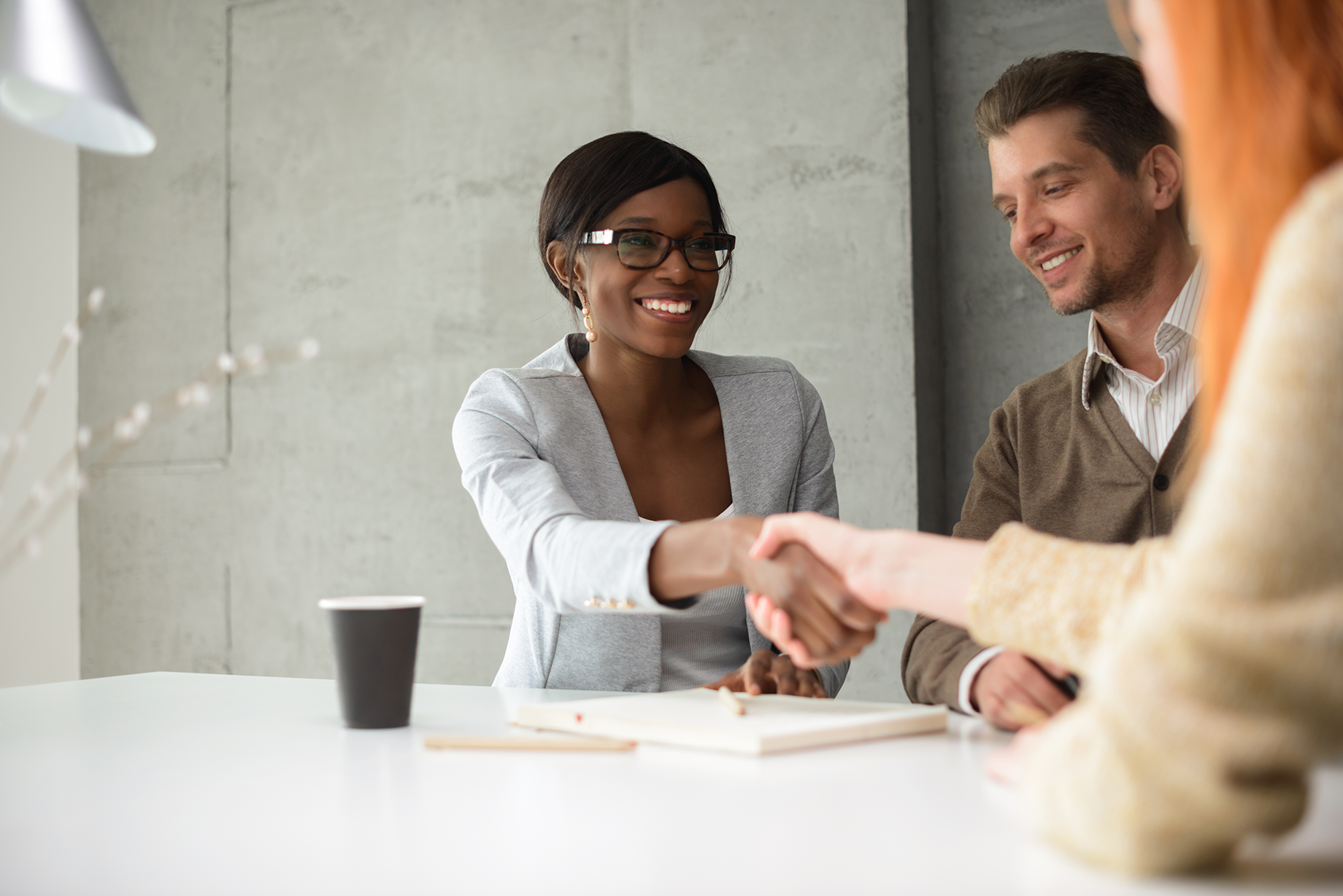
Better credit risk evaluation: How one lender improved its model by 50 percent
Published 25th May 2018Much of the public views consumer credit modeling and credit scores as tools to help lenders avoid customers that will default.
To a lender, credit scores are a little more complex. Credit scores don’t actually predict if a particular borrower will default. Instead, they rank borrowers according to a likelihood of default. A bank might lend to thousands of people with a credit score of 750. And thousands of people with a relatively poor credit score of 630. There will be defaults in both groups, but we’d expect to see more in the group with poor credit scores. Credit scores then, are really a model of risk.
But there is more than one way they can be wrong. First, they could assign less risk to borrowers who are likely to default — lumping the person with a 630 score in the 750s. Second, it could assign more risk to customers who are unlikely to default, which means they put low risk borrowers with 750 credit scores in the same group as high risk borrowers with 630 scores.
Both affect profitability. One would lead to a rise in the rate of unanticipated defaults. The other leads to the rejection of otherwise profitable customers – either by the lender itself, or because it offered too high an interest rate.
The accuracy of credit scores and other consumer credit models, therefore, can have a significant impact on the profitability of a lender.
Powerlytics recently conducted a pilot study with a customer to examine its ability to improve the accuracy of consumer credit risk evaluation at the individual or portfolio level.
Powerlytics developed a consumer default score by combining its database, which contains a comprehensive and anonymized financial view of over 200 million adults, with a loan-level customer data file of funded loans.
The results showed that with Powerlytics data, the customer could improve the accuracy of its model by approximately 50 percent, allowing it to better identify both risky clients and good customers.
To perform the analysis, we built a dataset that contained the customer’s loan data, along with a year of loan origination, certain relevant characteristics, and the customer’s geographic location down to the ZIP+4 level.
We then evaluated the data to identify variables that best predict default. Variables relating to an individual’s total federal tax due amount as a percentage of household income, adjusted gross income, and disposable income were among the set of Powerlytics variables that were most predictive of default.
Also, variables that relate to long-term savings in retirement accounts and pensions and annuities were significant predictors. Social security benefits amount (taxable) as a percentage of disposable income and adjusted gross income also contributed toward predicting default.
Interestingly, various income metrics (e.g., business income) of a ZIP+4 in which a borrower resides relative to the broader geography (zip code, state, etc.) were among significant predictors as well.
Powerlytics data, which covers a wide range of income and other economic and demographic items, has consistently outperformed or augmented the mix of demographic and financial information supplied by applicants themselves and credit bureaus.
When evaluated over time, Powerlytics Consumer Default Models can also detect signals that indicate credit risk in portfolios, enabling improved investment decision-making.
Whether using the data at the portfolio level or the consumer loan level, lenders can enhance their loan pricing, enticing borrowers with good credit, increasing their credit lines, or requiring less collateral before making a loan.
Conversely, the potential savings that can be had by anticipating defaults can be quite large.
Credit scores need to be accurate in more ways than one. With Powerlytics data, businesses can see a more accurate picture of their customers.
Read next
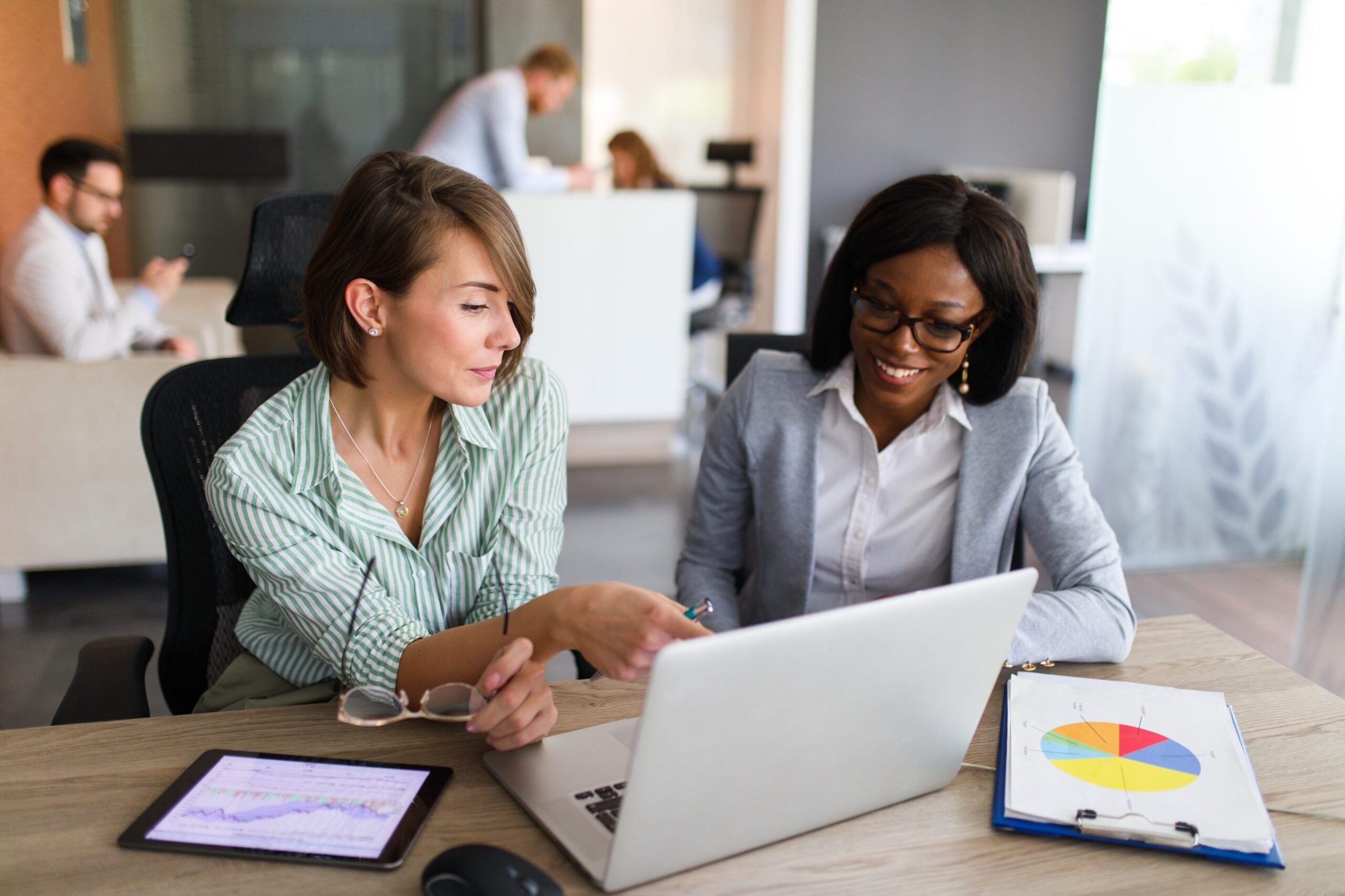
Published 23rd Jan 2018
MarketingOne data source takes on two challenges: Customer targeting and income verification
For lenders, finding people and businesses asking to borrow money isn’t much of a challenge. Finding businesses that can pay it back — that’s another story. For banks and alternative lenders that...
Read Article